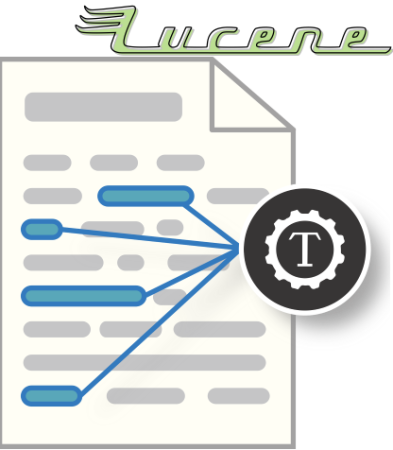
Nowadays, there are a lot of unstructured data available on the Internet, and more commonly, in Data Lakes (DL) specifically designed for Business Intelligence (BI). One of the main challenges in such Big Data environments is to find all similar documents which have common information.
To handle the challenge of finding similar free-text documents, there is a need to apply a structured text-mining process to execute two tasks: 1. profile the documents to extract their descriptive metadata, 2. to compare the profiles of pairs of documents to detect their overall similarity. Both tasks can be handled by an open-source text-mining project like Apache Lucene.
In Apache Lucene, there are supported capabilities to extract the descriptive metadata about the text-documents using frequent-terms extraction, then, for the second part of similarity computation, the project supports cosine-similarity computations between the TF-IDF terms frequencies (a popular information retrieval technique for free-text) for profiling the free-text documents. In laymen’s terms, this translates to the following process:
1. Create a new Apache Lucene index for the documents you will search for similarity
2. Add each document to the index
3. Profile each document in the index by extracting its frequent terms (usually modeled as a term vector where each term is accompanied with its frequency indicator inside the same document)
4. Compute the TF-IDF weighting-scheme of the frequent-terms for each document
5. Compute the cosine-similarity score between each pair of documents (returns a value between 0 and 1, where 0 means most different and 1 means most similar)
The TF-IDF is a very popular information retrieval technique for free-text documents. It weights the importance of each term in the whole corpus of documents, where repetitive terms in the corpus get less weight and where more unique and infrequent terms in the corpus get higher weights. This allows to give an importance for each term so that very common words like “and”, “to”, “from”, etc. do not get high importance in the similarity score computation when comparing a pair of documents as much as unique words like “biodiversity”, “algorithm”, “DNA-Microarray”, etc.
The Apache Lucene Java-based library (an easily integrated API with Maven-based Java projects) already includes an open-source implementation of the TF-IDF computation mechanisms and the cosine similarity scoring between documents. It includes multiple pre-processing functionality which can cleanse and prepare the documents for the text-mining process as follows:
· Tokenization: splitting the parts of the document to extract each term individually.
· Stemming: converting the words in a language to its simplest form or stem (e.g. in English, a word like “running” will be stemmed to “run”).
· Stop-word removal: removing the frequent repetitive words from the analysis which don’t matter in our similarity computation (e.g. common stop-words like “a”, “to”, etc.)
We demonstrate an example of utilizing this library using a sample of code below, where important lines of code are commented accordingly for emphasizing the task executed in the commented lines.
//Import the Java utility classes
import java.io.BufferedReader;
import java.io.FileReader;
import java.io.PrintWriter;
import java.util.ArrayList;
import java.util.HashMap;
import java.util.HashSet;
import java.util.Map;
import java.util.Set;
//Import the Apache Commons Maths library classes to handle the term-vectors from Apache Lucene
import org.apache.commons.math3.linear.ArrayRealVector;
import org.apache.commons.math3.linear.RealVector;
//Import the Apache Lucene classes
import org.apache.lucene.analysis.Analyzer;
import org.apache.lucene.analysis.core.SimpleAnalyzer;
import org.apache.lucene.document.Document;
import org.apache.lucene.document.Field;
import org.apache.lucene.document.FieldType;
import org.apache.lucene.index.AtomicReader;
import org.apache.lucene.index.DirectoryReader;
import org.apache.lucene.index.DocsEnum;
import org.apache.lucene.index.IndexReader;
import org.apache.lucene.index.IndexWriter;
import org.apache.lucene.index.IndexWriterConfig;
import org.apache.lucene.index.MultiFields;
import org.apache.lucene.index.Term;
import org.apache.lucene.index.Terms;
import org.apache.lucene.index.TermsEnum;
import org.apache.lucene.search.similarities.DefaultSimilarity;
import org.apache.lucene.search.similarities.TFIDFSimilarity;
import org.apache.lucene.store.Directory;
import org.apache.lucene.store.RAMDirectory;
import org.apache.lucene.util.Bits;
import org.apache.lucene.util.BytesRef;
import org.apache.lucene.util.Version;
import com.thoughtworks.xstream.core.util.Fields;
//A class to find the similarity of all text documents referenced in a text file
public class DocumentSimilarity {
private static final String CONTENT = "Content"; /*the name of the field stored by Apache Lucene which includes the text from the
text documents.*/
private Set<String> terms = new HashSet<>(); //changed state with new terms after each call to getTermFrequencies() method
private ArrayList<Integer> directoryIndex; //The Apache Lucene index for documents to mine for similarities
/* Indexed, tokenized, stored. */
public final FieldType TYPE_STORED = new FieldType(); //This is the field profiled by Apache Lucene to collect its term frequencies
private HashMap<String,Integer> termsCount; //stores the counts of all terms found in the corpus
private ArrayList<Integer> scannedDocs; //stores the IDs of docs already scanned and stored in termsCount
DocumentSimilarity(String lookupFile) {
//Initialise the field to profile in each document
TYPE_STORED.setIndexed(true);
TYPE_STORED.setTokenized(true);
TYPE_STORED.setStored(true);
TYPE_STORED.setStoreTermVectors(true);
TYPE_STORED.setStoreTermVectorPositions(true);
TYPE_STORED.freeze();
termsCount = new HashMap<String,Integer>();
scannedDocs = new ArrayList<Integer>();
try {
//initialise an in-memory (RAM-based) index of the documents in the input folder using Apache Lucene
Directory directory = createIndex(lookupFile);
IndexReader reader = DirectoryReader.open(directory);
String outputFile = "C:/Users/default/test/docs_similarity.csv"; /* the output file where the document similarities will be stored */
PrintWriter writer;
writer = new PrintWriter(outputFile, "UTF-8");
writer.println("doc1,doc2,similarity");
//loop on the documents in the folder and compare them together
for (int i=0; i<directoryIndex.size(); i++) {
for (int j=i+1; j<directoryIndex.size(); j++) {
Map<String, Double> f1 = getTermFrequencies(reader, i); //get the term frequencies profile of the first document
RealVector v1 = toRealVector(f1); //convert term frequencies profile to a vector
Map<String, Double> f2 = getTermFrequencies2(reader, j); //get the term frequencies profile of the second document
RealVector v2 = toRealVector(f2); //convert term frequencies profile to a vector
double sim = getCosineSimilarity(v1, v2); //compute the cosine similarity of the documents pair using their terms frequencies profiles
writer.println(directoryIndex.get(i)+","+directoryIndex.get(j)+","+sim); //write the similarity to an output CSV file
}
terms = new HashSet<>();
}
reader.close();
writer.close();
} catch (Exception e) {
e.printStackTrace();
}
writeTermsCount();
}
//A method to initialise an in-memory (RAM-based) index of the documents in the input file using Apache Lucene
Directory createIndex(String lookupFile) {
try {
Directory directory = new RAMDirectory();
/* Initialize the analyzer which profiles the text inside the documents. This analyser does all the pre-processing of the
* text including stop-word removal, tokenization, etc. depending on the type of analyzer used.
* Check the reference website for different types of analyzers: https://www.tutorialspoint.com/lucene/lucene_analysis.htm
*/
Analyzer analyzer = new SimpleAnalyzer(Version.LUCENE_CURRENT); //create a new SimpleAnalyzer
IndexWriterConfig iwc = new IndexWriterConfig(Version.LUCENE_CURRENT,
analyzer);
IndexWriter writer = new IndexWriter(directory, iwc); //write the index of the documents with their analysis in-memory
int did=1; //documents are numbered by a document ID as 1,2,3...n in a lookup file of the documents to be indexed
BufferedReader dsLookup;
dsLookup = new BufferedReader(new FileReader(lookupFile));
String ds; //The document number in each line
ArrayList<Integer> directoryIndex = new ArrayList<Integer>();
while ((ds = dsLookup.readLine()) != null) {
//Set the document id to the string read from the line in lookup file
try {
String documentText = ""; //stores the text from the document
FileReader fr = new FileReader(did+".txt");
BufferedReader br = new BufferedReader(fr);
String sCurrentLine;
while ((sCurrentLine = br.readLine()) != null) {
documentText += (sCurrentLine);
}
//add the document to the index
addDocument(writer, documentText);
directoryIndex.add(did);
} catch (Exception e) {
System.out.println("!!! Document "+did+" has an error and was skipped !!!");
continue;
}
}
writer.close();
this.directoryIndex = directoryIndex;
return directory;
} catch (Exception e) {
e.printStackTrace();
}
return null;
}
//a method to add a document to the index by writing its text as a "content" field in Apache Lucene
void addDocument(IndexWriter writer, String content) {
try {
Document doc = new Document(); //create a new Apache Lucene document
Field field = new Field(CONTENT, content, TYPE_STORED); //Add the field with the text content to the index
doc.add(field);
writer.addDocument(doc);
} catch (Exception e) {
e.printStackTrace();
}
}
//a method to get the cosine similarity between two term-frequency vectors from a pair of documents
public double getCosineSimilarity(RealVector v1, RealVector v2) {
return (v1.dotProduct(v2)) / (v1.getNorm() * v2.getNorm());
}
//a method to get the term frequencies from a document
Map<String, Double> getTermFrequencies(IndexReader reader, int docId) {
try {
Terms vector = reader.getTermVector(docId, CONTENT);
TermsEnum termsEnum = null;
termsEnum = vector.iterator(termsEnum);
Map<String, Double> frequencies = new HashMap<>();
BytesRef text = null;
TFIDFSimilarity tfidfSim = new DefaultSimilarity();
boolean scannedDoc = scannedDocs.contains(docId);
while ((text = termsEnum.next()) != null) {
String term = text.utf8ToString();
org.apache.lucene.index.Fields fields = reader.getTermVectors(0);
Term termInstance = new Term("Content", term);
long indexDf = reader.docFreq(termInstance);
int docCount = reader.numDocs();
//increment the term count in the terms count lookup if doc not scanned before
if(!scannedDoc) {
if(termsCount.containsKey(termInstance.toString())) {
Integer cnt = termsCount.get(termInstance.toString());
cnt++;
termsCount.replace(termInstance.toString(), cnt);
} else {
termsCount.put(termInstance.toString(), 1);
}
}
DocsEnum docs = termsEnum.docs(MultiFields.getLiveDocs(reader),null,0);
//calculate the TF-IDF of the term, as compared to all documents in the corpus (the Apache Lucene Index)
double tfidf = 0.0;
while(docs.nextDoc() != DocsEnum.NO_MORE_DOCS) {
tfidf = tfidfSim.tf(docs.freq()) * tfidfSim.idf(docCount, indexDf);
}
frequencies.put(term, tfidf);
scannedDocs.add(docId);
terms.add(term);
}
return frequencies;
} catch (Exception e) {
e.printStackTrace();
}
return null;
}
Map<String, Double> getTermFrequencies2(IndexReader reader, int docId) {
try {
Terms vector = reader.getTermVector(docId, CONTENT);
TermsEnum termsEnum = null;
termsEnum = vector.iterator(termsEnum);
Map<String, Double> frequencies = new HashMap<>();
BytesRef text = null;
TFIDFSimilarity tfidfSim = new DefaultSimilarity();
boolean scannedDoc = scannedDocs.contains(docId);
int docCount = reader.numDocs();
while ((text = termsEnum.next()) != null) {
String term = text.utf8ToString();
Term termInstance = new Term("Content", term);
long indexDf = reader.docFreq(termInstance);
//increment the term count in the terms count lookup if doc not scanned before
if(!scannedDoc) {
if(termsCount.containsKey(termInstance.toString())) {
Integer cnt = termsCount.get(termInstance.toString());
cnt++;
termsCount.replace(termInstance.toString(), cnt);
} else {
termsCount.put(termInstance.toString(), 1);
}
}
DocsEnum docs = termsEnum.docs(MultiFields.getLiveDocs(reader),null,0);
double tfidf = 0.0;
while(docs.nextDoc() != DocsEnum.NO_MORE_DOCS) {
tfidf = tfidfSim.tf(docs.freq()) * tfidfSim.idf(docCount, indexDf);
}
frequencies.put(term, tfidf);
scannedDocs.add(docId);
}
return frequencies;
} catch (Exception e) {
e.printStackTrace();
}
return null;
}
//convert the term-frequencies extracted to a real vector
RealVector toRealVector(Map<String, Double> map) {
RealVector vector = new ArrayRealVector(terms.size());
int i = 0;
for (String term : terms) {
double value = map.containsKey(term) ? map.get(term) : 0.0;
vector.setEntry(i++, value);
}
return (RealVector) vector.mapDivide(vector.getL1Norm());
}
//Write the terms-frequencies from the documents to a CSV file
private void writeTermsCount() {
try {
String outputFile = "C:/Users/default/test/document_terms_count.csv";
PrintWriter writer;
writer = new PrintWriter(outputFile, "UTF-8");
writer.println("term,count");
for (String key : termsCount.keySet()) {
writer.println(key+","+termsCount.get(key));
}
writer.close();
} catch (Exception e) {
e.printStackTrace();
}
}
//MAIN class to execute with the .txt-file lookup of the documents to index
public static void main(String[] args) {
new DocumentSimilarity("C:/Users/default/test/documents_lookup.txt");
}
}